Keeping an Eye on artificial Intelligence
Automatic and smart data analysis is needed in many areas. This is usually achieved using artificial intelligence, which in turn is often based on neural networks. However, what exactly these neural networks do in their decision-making processes is mostly unknown. Fraunhofer FHR is now investigating the processes that were previously still in the dark.
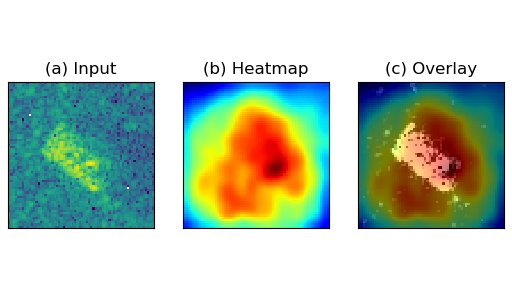
Artificial intelligence and neural networks are hot topics that run through numerous areas – from mobility and production processes through defense. After all, the data volumes collected are becoming increasingly large, so the support needed for their evaluation is growing steadily. Although the neural networks generally deliver good results, usually it is not known how they arrive at these results. The neural networks are similar to a black box. Sometimes they rely on unimportant information when performing an image classification task. For example, in one project described in the scientific literature which involved the classification of ships in optical images, the neural networks analyzed the water instead of the ships!
Do neural networks do what they are supposed to do? Are their results therefore reliable? Researchers at Fraunhofer FHR are investigating these questions under the heading of Explainable AI. The work is based on public radar data obtained during two overflights over a field. Ten targets were placed on the field beforehand – tanks, trucks, armored vehicles and a bulldozer. The radar data generated during the first overflight was used as training data. The test data was collected during the second overflight as the targets remained untouched and only the observation angle was modified. It would thus be entirely possible for the neural networks to classify the radar images by background, such as a tree standing next to the tank.
The analysis was carried out using both feature maps and heat maps. The algorithms they use are known, but they have not yet been applied to the analysis of radar data. Visualizing feature maps at each individual layer helps to understand what the neural network learns in order to make a specific decision or prediction. The focus here is on features such as edges or the length of the target. However, in deep neural networks, this type of analysis quickly becomes confusing. This reason is that the deeper the network gets, the more complex the feature map becomes. Therefore, all important features that the neural networks "look at" were combined in a heat map in a second analysis. The results validate Fraunhofer FHR's neural networks: As desired, they analyze the targets, not the background.